Quantifying Curb Appeal
Zachary Bessinger and Nathan Jacobs
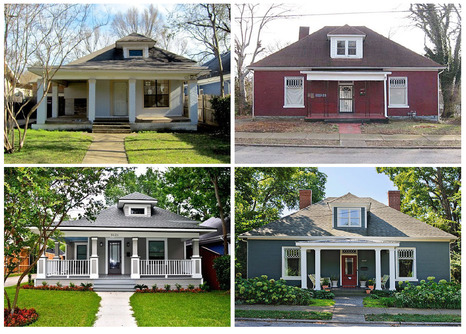
Abstract
The curb appeal of a home, which refers to how attractive it is when viewed from the street, is an important decision-making factor for many home buyers. Existing models for automatically estimating the price of a home ignore this factor, instead focusing exclusively on objective attributes, such as number of bedrooms, the square footage, and the age. We propose to use street-level imagery of a home, in addition to the objective attributes, to estimate the price of the home, thereby quantifying curb appeal. Our method uses deep convolutional neural networks to extract informative image features. We introduce a large dataset to support an extensive evaluation of several approaches. We find that using images and objective attributes together results in more accurate home price estimates than using either in isolation. We also find that representations learned for scene classification tasks are more discriminative for home-price estimation than those learned for other tasks.
Downloads
Paper (pdf) Poster (pdf) DatasetBibTeX
@inproceedings{bessinger2016quantifying, title = {Quantifying Curb Appeal}, author = {Bessinger, Zachary and Jacobs, Nathan}, booktitle = {IEEE International Conference on Image Processing (ICIP)}, year = {2016}, organization = {IEEE} }