Sky Segmentation in the Wild: An Empirical Study
Radu Mihail, Scott Workman, Zachary Bessinger, and Nathan Jacobs
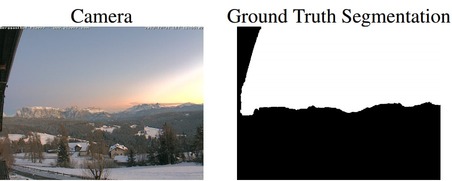
Abstract
Automatically determining which pixels in an image view the sky, the problem of sky segmentation, is a critical pre-processing step for a wide variety of outdoor image interpretation problems, including horizon estimation, robot navigation and image geolocalization. Many methods for this problem have been proposed with recent work achieving significant improvements on benchmark datasets. However, such datasets are often constructed to contain images captured in favorable conditions and, therefore, do not reflect the broad range of conditions with which a real-world vision system must cope. This paper presents the results of a large-scale empirical evaluation of the performance of three state-of-the-art approaches on a new dataset, which consists of roughly 100k images captured "in the wild". The results show that the performance of these methods can be dramatically degraded by the local lighting and weather conditions. We propose a deep learning based variant of an ensemble solution that outperform the methods we tested, in some cases achieving above 50% relative reduction in misclassified pixels. While our results show there is room for improvement, our hope is that this dataset will encourage others to improve the real-world performance of their algorithms.
Downloads
Paper (pdf)BibTeX
@inproceedings{mihail2016sky, title = {Sky Segmentation in the Wild: An Empirical Study}, author = {Mihail, Radu and Workman, Scott and Bessinger, Zachary and Jacobs, Nathan}, booktitle = {IEEE Winter Conference on Applications of Computer Vision (WACV)}, year = {2016}, organization = {IEEE} }